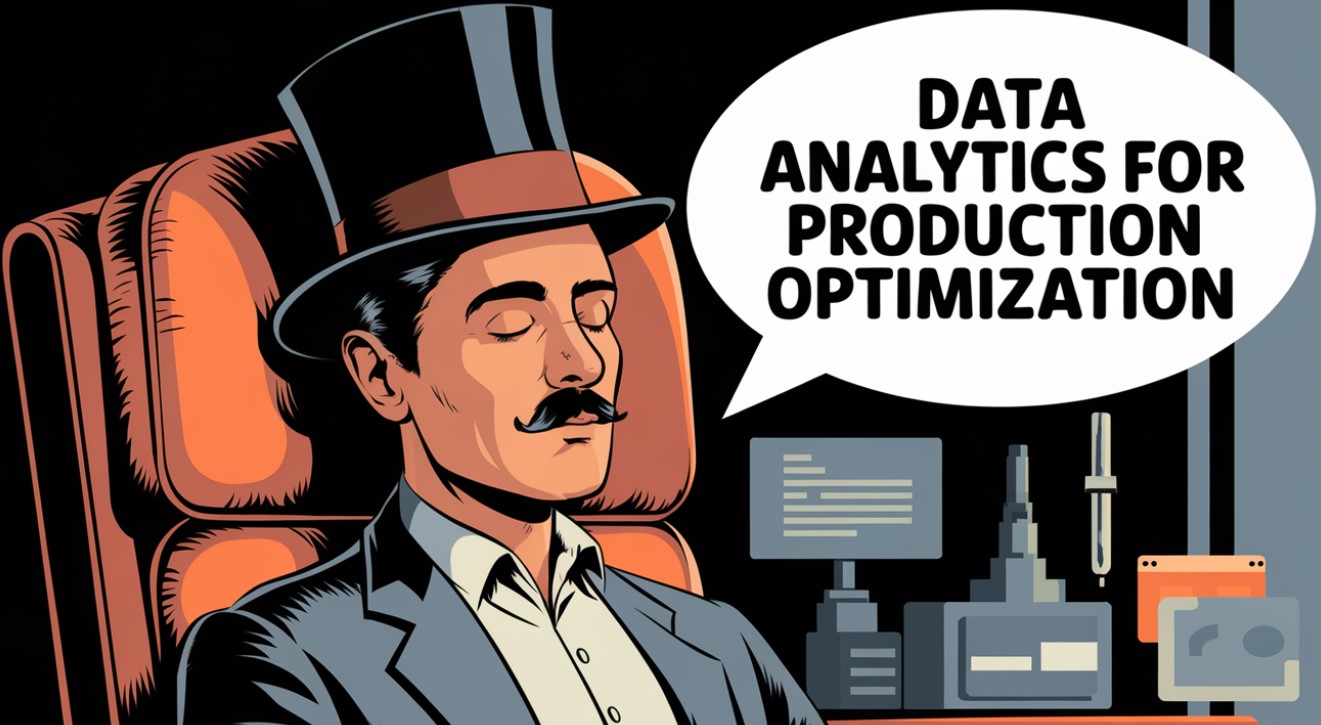
Picture your production line as a perfectly running machine. What if there were ways to achieve even better results, accelerating the journey toward greater efficiency? That’s exactly where data analytics comes into play. By leveraging insights from data, organizations can move faster, smarter, and more strategically. It is almost like a diagnostic tool that finds hidden inefficiencies and potential areas of improvement. This guide details the step-by-step application of data analytics to optimize production in a friendly and actionable manner.
1. Define Your Goals: What Problem Are You Trying to Solve?
Before one gets into data, the most significant consideration is to think about what one wants to attain. What objectives might you have? Would it be
- Reduced downtime?
- Increased throughput?
- Improved quality?
- Less waste?
- Optimized energy consumption?
Setting your objectives narrows the scope of work and provides easy access to outcome measurements. For instance, instead of saying improve efficiency, say reduce machine downtime by 15% within six months.
For more information: Business Ideas and Investment Opportunities
2. Gather Your Data: The Raw Material of Optimization
Every day on the production floor, vast amounts of data are generated—data that can fuel innovation and boost efficiency. It’s remarkable how much actionable information can emerge from just a single day’s operations. Some include:
- Sensor data: Temperature, pressure, vibration, etc.
- Machine logs: operating parameters, error codes, and maintenance records.
- Production records: output, cycle times, and defect rates.
- Quality control data: inspection results, customer feedback.
It is critical to collect data on things relevant to your objectives. Make sure your data is valid, consistent, and stored in a format that allows analysis easily. You may need to invest in sensors, data loggers, or data management systems to make sure you have exactly what you need.
3. Clean and Prepare Your Data: Taming the Wild West
Raw data is rarely good enough. Most of the time, it has errors, missing values, or inconsistencies. Data cleaning is the process of converting raw data into a usable format. The fundamentals of this are
- Removing duplicates: Making sure each data point remains unique.
- Dealing with missing values: filling in the blanks or deleting incomplete records.
- Standardizing formats: Ensuring uniformity in data when coming from different sources.
- Identifying and removing outliers: Detecting and remedying unusual data points.
This procedure is of utmost importance to ensure accurate and reliable analysis. Think of it like preparing your ingredients before cooking: an essential part of any truly wonderful product.
4. Analyze Your Data: Bringing Insights to the Fore
Your data deserves to be transformed into actionable insights now. Whatever analytical methods you adopt will depend on your objectives.
- Descriptive analytics: A form of data summarization to capture insight into current-future performance. The average cycle time and defect rates will be calculated.
- Diagnostic analytics: Sorting through seemingly unrelated circumstances to determine the responsible causative factor(s). Use correlation analysis, for instance, to know how machine parameters tie with defect rates.
- Predictive analytics: Used to predict future performance. Machine learning could therefore be applied here to predict failure of a machine.
- Prescriptive analytics: Suitable to provide recommendations to improve or optimize performance. In this example, we could recommend a particular setting on the machine to reduce energy consumption.
To perform these analyses, you could choose among spreadsheets, statistical tools, and data graphics software.
5. Visualize Your Findings: Making Data Understandable
Data visualization is such that it would reduce complex data into intelligible insights. Consider a foreign language-it is translated into the native tongue. It shows specific trends of downtimes in line charts, temperature-quality relationships through scatter plots, and today-time overviews on dashboards.
Visuals help users quickly spot patterns and anomalies, enabling faster and more informed decisions. By transforming raw data into intuitive graphics, visualizations empower stakeholders across the organization to better understand key insights. This fosters collaboration and drives informed actions. When data tells a story through visuals, it becomes more accessible and meaningful to everyone, making it a powerful tool for effective optimization.
In making changes, it should ideally be done in a controlled manner while observing how these changes affect the process.
7. Monitor and Iterate: Continuous Improvement
Data analytics is a continuing endeavour. You will have to keep watch over and make iterations to your improvement efforts of the production processes. That means:
- Having a look at key performance indicators (KPIs): To measure the impact of your changes.
- Collecting new data: To remain current regarding the state of your production processes.
- Refining your analysis: Altering your approach to match.
Through continuous monitoring and iteration, you will ensure that your production process will always improve.
Youtube link: Entrepreneur India
Closing Thoughts
With data analytics, you will be empowered to make data-driven decisions that can drastically change the way you utilize production processes. It is about converting what is known information into tactical knowledge. With this guideline, you will be able to pry the hidden power away from production data, thereby providing great returns in efficiency, quality, and cost. This continuous improvement journey that you have embarked on is well worth it.